mirror of
https://github.com/blakeblackshear/frigate.git
synced 2025-07-30 13:48:07 +02:00
commit
3e803b6a03
37
Dockerfile
37
Dockerfile
@ -26,23 +26,29 @@ RUN apt-get -qq update && apt-get -qq install --no-install-recommends -y python3
|
|||||||
vim \
|
vim \
|
||||||
ffmpeg \
|
ffmpeg \
|
||||||
unzip \
|
unzip \
|
||||||
|
libusb-1.0-0-dev \
|
||||||
|
python3-setuptools \
|
||||||
|
python3-numpy \
|
||||||
|
zlib1g-dev \
|
||||||
|
libgoogle-glog-dev \
|
||||||
|
swig \
|
||||||
|
libunwind-dev \
|
||||||
|
libc++-dev \
|
||||||
|
libc++abi-dev \
|
||||||
|
build-essential \
|
||||||
&& rm -rf /var/lib/apt/lists/*
|
&& rm -rf /var/lib/apt/lists/*
|
||||||
|
|
||||||
# Install core packages
|
# Install core packages
|
||||||
RUN wget -q -O /tmp/get-pip.py --no-check-certificate https://bootstrap.pypa.io/get-pip.py && python3 /tmp/get-pip.py
|
RUN wget -q -O /tmp/get-pip.py --no-check-certificate https://bootstrap.pypa.io/get-pip.py && python3 /tmp/get-pip.py
|
||||||
RUN pip install -U pip \
|
RUN pip install -U pip \
|
||||||
numpy \
|
numpy \
|
||||||
|
pillow \
|
||||||
matplotlib \
|
matplotlib \
|
||||||
notebook \
|
notebook \
|
||||||
jupyter \
|
|
||||||
pandas \
|
|
||||||
moviepy \
|
|
||||||
tensorflow \
|
|
||||||
keras \
|
|
||||||
autovizwidget \
|
|
||||||
Flask \
|
Flask \
|
||||||
imutils \
|
imutils \
|
||||||
paho-mqtt
|
paho-mqtt \
|
||||||
|
PyYAML
|
||||||
|
|
||||||
# Install tensorflow models object detection
|
# Install tensorflow models object detection
|
||||||
RUN GIT_SSL_NO_VERIFY=true git clone -q https://github.com/tensorflow/models /usr/local/lib/python3.5/dist-packages/tensorflow/models
|
RUN GIT_SSL_NO_VERIFY=true git clone -q https://github.com/tensorflow/models /usr/local/lib/python3.5/dist-packages/tensorflow/models
|
||||||
@ -59,9 +65,6 @@ RUN cd /usr/local/src/ \
|
|||||||
&& ldconfig \
|
&& ldconfig \
|
||||||
&& rm -rf /usr/local/src/protobuf-3.5.1/
|
&& rm -rf /usr/local/src/protobuf-3.5.1/
|
||||||
|
|
||||||
# Add dataframe display widget
|
|
||||||
RUN jupyter nbextension enable --py --sys-prefix widgetsnbextension
|
|
||||||
|
|
||||||
# Download & build OpenCV
|
# Download & build OpenCV
|
||||||
RUN wget -q -P /usr/local/src/ --no-check-certificate https://github.com/opencv/opencv/archive/4.0.1.zip
|
RUN wget -q -P /usr/local/src/ --no-check-certificate https://github.com/opencv/opencv/archive/4.0.1.zip
|
||||||
RUN cd /usr/local/src/ \
|
RUN cd /usr/local/src/ \
|
||||||
@ -75,10 +78,24 @@ RUN cd /usr/local/src/ \
|
|||||||
&& make install \
|
&& make install \
|
||||||
&& rm -rf /usr/local/src/opencv-4.0.1
|
&& rm -rf /usr/local/src/opencv-4.0.1
|
||||||
|
|
||||||
|
# Download and install EdgeTPU libraries
|
||||||
|
RUN wget -q -O edgetpu_api.tar.gz --no-check-certificate http://storage.googleapis.com/cloud-iot-edge-pretrained-models/edgetpu_api.tar.gz
|
||||||
|
|
||||||
|
RUN tar xzf edgetpu_api.tar.gz \
|
||||||
|
&& cd python-tflite-source \
|
||||||
|
&& cp -p libedgetpu/libedgetpu_x86_64.so /lib/x86_64-linux-gnu/libedgetpu.so \
|
||||||
|
&& cp edgetpu/swig/compiled_so/_edgetpu_cpp_wrapper_x86_64.so edgetpu/swig/_edgetpu_cpp_wrapper.so \
|
||||||
|
&& cp edgetpu/swig/compiled_so/edgetpu_cpp_wrapper.py edgetpu/swig/ \
|
||||||
|
&& python3 setup.py develop --user
|
||||||
|
|
||||||
# Minimize image size
|
# Minimize image size
|
||||||
RUN (apt-get autoremove -y; \
|
RUN (apt-get autoremove -y; \
|
||||||
apt-get autoclean -y)
|
apt-get autoclean -y)
|
||||||
|
|
||||||
|
# symlink the model and labels
|
||||||
|
RUN ln -s /python-tflite-source/edgetpu/test_data/mobilenet_ssd_v2_coco_quant_postprocess_edgetpu.tflite /frozen_inference_graph.pb
|
||||||
|
RUN ln -s /python-tflite-source/edgetpu/test_data/coco_labels.txt /label_map.pbtext
|
||||||
|
|
||||||
# Set TF object detection available
|
# Set TF object detection available
|
||||||
ENV PYTHONPATH "$PYTHONPATH:/usr/local/lib/python3.5/dist-packages/tensorflow/models/research:/usr/local/lib/python3.5/dist-packages/tensorflow/models/research/slim"
|
ENV PYTHONPATH "$PYTHONPATH:/usr/local/lib/python3.5/dist-packages/tensorflow/models/research:/usr/local/lib/python3.5/dist-packages/tensorflow/models/research/slim"
|
||||||
RUN cd /usr/local/lib/python3.5/dist-packages/tensorflow/models/research && protoc object_detection/protos/*.proto --python_out=.
|
RUN cd /usr/local/lib/python3.5/dist-packages/tensorflow/models/research && protoc object_detection/protos/*.proto --python_out=.
|
||||||
|
119
README.md
119
README.md
@ -1,18 +1,18 @@
|
|||||||
# Frigate - Realtime Object Detection for RTSP Cameras
|
# Frigate - Realtime Object Detection for RTSP Cameras
|
||||||
|
**Note:** This version requires the use of a [Google Coral USB Accelerator](https://coral.withgoogle.com/products/accelerator/)
|
||||||
|
|
||||||
Uses OpenCV and Tensorflow to perform realtime object detection locally for RTSP cameras. Designed for integration with HomeAssistant or others via MQTT.
|
Uses OpenCV and Tensorflow to perform realtime object detection locally for RTSP cameras. Designed for integration with HomeAssistant or others via MQTT.
|
||||||
|
|
||||||
- Leverages multiprocessing and threads heavily with an emphasis on realtime over processing every frame
|
- Leverages multiprocessing and threads heavily with an emphasis on realtime over processing every frame
|
||||||
- Allows you to define specific regions (squares) in the image to look for motion/objects
|
- Allows you to define specific regions (squares) in the image to look for objects
|
||||||
- Motion detection runs in a separate process per region and signals to object detection to avoid wasting CPU cycles looking for objects when there is no motion
|
- No motion detection (for now)
|
||||||
- Object detection with Tensorflow runs in a separate process per region
|
- Object detection with Tensorflow runs in a separate thread
|
||||||
- Detected objects are placed on a shared mp.Queue and aggregated into a list of recently detected objects in a separate thread
|
- Object info is published over MQTT for integration into HomeAssistant as a binary sensor
|
||||||
- A person score is calculated as the sum of all scores/5
|
|
||||||
- Motion and object info is published over MQTT for integration into HomeAssistant or others
|
|
||||||
- An endpoint is available to view an MJPEG stream for debugging
|
- An endpoint is available to view an MJPEG stream for debugging
|
||||||
|
|
||||||

|

|
||||||
|
|
||||||
## Example video
|
## Example video (from older version)
|
||||||
You see multiple bounding boxes because it draws bounding boxes from all frames in the past 1 second where a person was detected. Not all of the bounding boxes were from the current frame.
|
You see multiple bounding boxes because it draws bounding boxes from all frames in the past 1 second where a person was detected. Not all of the bounding boxes were from the current frame.
|
||||||
[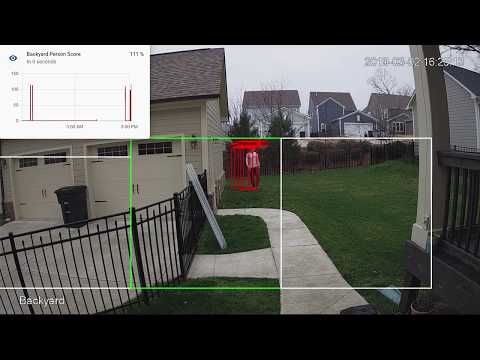](http://www.youtube.com/watch?v=nqHbCtyo4dY "Frigate")
|
[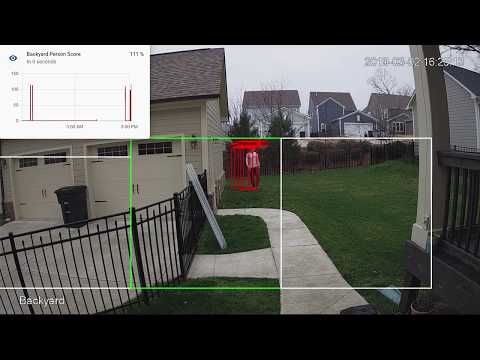](http://www.youtube.com/watch?v=nqHbCtyo4dY "Frigate")
|
||||||
|
|
||||||
@ -22,24 +22,16 @@ Build the container with
|
|||||||
docker build -t frigate .
|
docker build -t frigate .
|
||||||
```
|
```
|
||||||
|
|
||||||
Download a model from the [zoo](https://github.com/tensorflow/models/blob/master/research/object_detection/g3doc/detection_model_zoo.md).
|
The `mobilenet_ssd_v2_coco_quant_postprocess_edgetpu.tflite` model is included and used by default. You can use your own model and labels by mounting files in the container at `/frozen_inference_graph.pb` and `/label_map.pbtext`. Models must be compatible with the Coral according to [this](https://coral.withgoogle.com/models/).
|
||||||
|
|
||||||
Download the cooresponding label map from [here](https://github.com/tensorflow/models/tree/master/research/object_detection/data).
|
|
||||||
|
|
||||||
Run the container with
|
Run the container with
|
||||||
```
|
```
|
||||||
docker run --rm \
|
docker run --rm \
|
||||||
-v <path_to_frozen_detection_graph.pb>:/frozen_inference_graph.pb:ro \
|
--privileged \
|
||||||
-v <path_to_labelmap.pbtext>:/label_map.pbtext:ro \
|
-v /dev/bus/usb:/dev/bus/usb \
|
||||||
-v <path_to_config_dir>:/config:ro \
|
-v <path_to_config_dir>:/config:ro \
|
||||||
-p 5000:5000 \
|
-p 5000:5000 \
|
||||||
-e RTSP_URL='<rtsp_url>' \
|
-e RTSP_PASSWORD='password' \
|
||||||
-e REGIONS='<box_size_1>,<x_offset_1>,<y_offset_1>,<min_person_size_1>,<min_motion_size_1>,<mask_file_1>:<box_size_2>,<x_offset_2>,<y_offset_2>,<min_person_size_2>,<min_motion_size_2>,<mask_file_2>' \
|
|
||||||
-e MQTT_HOST='your.mqtthost.com' \
|
|
||||||
-e MQTT_USER='username' \
|
|
||||||
-e MQTT_PASS='password' \
|
|
||||||
-e MQTT_TOPIC_PREFIX='cameras/1' \
|
|
||||||
-e DEBUG='0' \
|
|
||||||
frigate:latest
|
frigate:latest
|
||||||
```
|
```
|
||||||
|
|
||||||
@ -48,100 +40,59 @@ Example docker-compose:
|
|||||||
frigate:
|
frigate:
|
||||||
container_name: frigate
|
container_name: frigate
|
||||||
restart: unless-stopped
|
restart: unless-stopped
|
||||||
|
privileged: true
|
||||||
image: frigate:latest
|
image: frigate:latest
|
||||||
volumes:
|
volumes:
|
||||||
- <path_to_frozen_detection_graph.pb>:/frozen_inference_graph.pb:ro
|
- /dev/bus/usb:/dev/bus/usb
|
||||||
- <path_to_labelmap.pbtext>:/label_map.pbtext:ro
|
|
||||||
- <path_to_config>:/config
|
- <path_to_config>:/config
|
||||||
ports:
|
ports:
|
||||||
- "127.0.0.1:5000:5000"
|
- "5000:5000"
|
||||||
environment:
|
environment:
|
||||||
RTSP_URL: "<rtsp_url>"
|
RTSP_PASSWORD: "password"
|
||||||
REGIONS: "<box_size_1>,<x_offset_1>,<y_offset_1>,<min_person_size_1>,<min_motion_size_1>,<mask_file_1>:<box_size_2>,<x_offset_2>,<y_offset_2>,<min_person_size_2>,<min_motion_size_2>,<mask_file_2>"
|
|
||||||
MQTT_HOST: "your.mqtthost.com"
|
|
||||||
MQTT_USER: "username" #optional
|
|
||||||
MQTT_PASS: "password" #optional
|
|
||||||
MQTT_TOPIC_PREFIX: "cameras/1"
|
|
||||||
DEBUG: "0"
|
|
||||||
```
|
```
|
||||||
|
|
||||||
Here is an example `REGIONS` env variable:
|
A `config.yml` file must exist in the `config` directory. See example [here](config/config.yml).
|
||||||
`350,0,300,5000,200,mask-0-300.bmp:400,350,250,2000,200,mask-350-250.bmp:400,750,250,2000,200,mask-750-250.bmp`
|
|
||||||
|
|
||||||
First region broken down (all are required):
|
Access the mjpeg stream at `http://localhost:5000/<camera_name>` and the best person snapshot at `http://localhost:5000/<camera_name>/best_person.jpg`
|
||||||
- `350` - size of the square (350px by 350px)
|
|
||||||
- `0` - x coordinate of upper left corner (top left of image is 0,0)
|
|
||||||
- `300` - y coordinate of upper left corner (top left of image is 0,0)
|
|
||||||
- `5000` - minimum person bounding box size (width*height for bounding box of identified person)
|
|
||||||
- `200` - minimum number of changed pixels to trigger motion
|
|
||||||
- `mask-0-300.bmp` - a bmp file with the masked regions as pure black, must be the same size as the region
|
|
||||||
|
|
||||||
Mask files go in the `/config` directory.
|
|
||||||
|
|
||||||
Access the mjpeg stream at http://localhost:5000
|
|
||||||
|
|
||||||
## Integration with HomeAssistant
|
## Integration with HomeAssistant
|
||||||
```
|
```
|
||||||
camera:
|
camera:
|
||||||
- name: Camera Last Person
|
- name: Camera Last Person
|
||||||
platform: generic
|
platform: generic
|
||||||
still_image_url: http://<ip>:5000/best_person.jpg
|
still_image_url: http://<ip>:5000/<camera_name>/best_person.jpg
|
||||||
|
|
||||||
binary_sensor:
|
|
||||||
- name: Camera Motion
|
|
||||||
platform: mqtt
|
|
||||||
state_topic: "cameras/1/motion"
|
|
||||||
device_class: motion
|
|
||||||
availability_topic: "cameras/1/available"
|
|
||||||
|
|
||||||
sensor:
|
sensor:
|
||||||
- name: Camera Person Score
|
- name: Camera Person
|
||||||
platform: mqtt
|
platform: mqtt
|
||||||
state_topic: "cameras/1/objects"
|
state_topic: "frigate/<camera_name>/objects"
|
||||||
value_template: '{{ value_json.person }}'
|
value_template: '{{ value_json.person }}'
|
||||||
unit_of_measurement: '%'
|
device_class: moving
|
||||||
availability_topic: "cameras/1/available"
|
availability_topic: "frigate/available"
|
||||||
```
|
```
|
||||||
|
|
||||||
## Tips
|
## Tips
|
||||||
- Lower the framerate of the RTSP feed on the camera to reduce the CPU usage for capturing the feed
|
- Lower the framerate of the RTSP feed on the camera to reduce the CPU usage for capturing the feed
|
||||||
- Use SSDLite models to reduce CPU usage
|
|
||||||
|
|
||||||
## Future improvements
|
## Future improvements
|
||||||
- [ ] Build tensorflow from source for CPU optimizations
|
- [x] Remove motion detection for now
|
||||||
|
- [x] Try running object detection in a thread rather than a process
|
||||||
|
- [x] Implement min person size again
|
||||||
|
- [x] Switch to a config file
|
||||||
|
- [x] Handle multiple cameras in the same container
|
||||||
|
- [ ] Attempt to figure out coral symlinking
|
||||||
|
- [ ] Add object list to config with min scores for mqtt
|
||||||
|
- [ ] Move mjpeg encoding to a separate process
|
||||||
|
- [ ] Simplify motion detection (check entire image against mask, resize instead of gaussian blur)
|
||||||
|
- [ ] See if motion detection is even worth running
|
||||||
|
- [ ] Scan for people across entire image rather than specfic regions
|
||||||
|
- [ ] Dynamically resize detection area and follow people
|
||||||
- [ ] Add ability to turn detection on and off via MQTT
|
- [ ] Add ability to turn detection on and off via MQTT
|
||||||
- [ ] MQTT motion occasionally gets stuck ON
|
|
||||||
- [ ] Output movie clips of people for notifications, etc.
|
- [ ] Output movie clips of people for notifications, etc.
|
||||||
- [ ] Integrate with homeassistant push camera
|
- [ ] Integrate with homeassistant push camera
|
||||||
- [ ] Merge bounding boxes that span multiple regions
|
- [ ] Merge bounding boxes that span multiple regions
|
||||||
- [ ] Switch to a config file
|
|
||||||
- [ ] Allow motion regions to be different than object detection regions
|
|
||||||
- [ ] Implement mode to save labeled objects for training
|
- [ ] Implement mode to save labeled objects for training
|
||||||
- [ ] Try and reduce CPU usage by simplifying the tensorflow model to just include the objects we care about
|
- [ ] Try and reduce CPU usage by simplifying the tensorflow model to just include the objects we care about
|
||||||
- [ ] Look into GPU accelerated decoding of RTSP stream
|
- [ ] Look into GPU accelerated decoding of RTSP stream
|
||||||
- [ ] Send video over a socket and use JSMPEG
|
- [ ] Send video over a socket and use JSMPEG
|
||||||
- [ ] Look into neural compute stick
|
- [x] Look into neural compute stick
|
||||||
|
|
||||||
## Building Tensorflow from source for CPU optimizations
|
|
||||||
https://www.tensorflow.org/install/source#docker_linux_builds
|
|
||||||
used `tensorflow/tensorflow:1.12.0-devel-py3`
|
|
||||||
|
|
||||||
## Optimizing the graph (cant say I saw much difference in CPU usage)
|
|
||||||
https://github.com/tensorflow/tensorflow/blob/master/tensorflow/tools/graph_transforms/README.md#optimizing-for-deployment
|
|
||||||
```
|
|
||||||
docker run -it -v ${PWD}:/lab -v ${PWD}/../back_camera_model/models/ssd_mobilenet_v2_coco_2018_03_29/frozen_inference_graph.pb:/frozen_inference_graph.pb:ro tensorflow/tensorflow:1.12.0-devel-py3 bash
|
|
||||||
|
|
||||||
bazel build tensorflow/tools/graph_transforms:transform_graph
|
|
||||||
|
|
||||||
bazel-bin/tensorflow/tools/graph_transforms/transform_graph \
|
|
||||||
--in_graph=/frozen_inference_graph.pb \
|
|
||||||
--out_graph=/lab/optimized_inception_graph.pb \
|
|
||||||
--inputs='image_tensor' \
|
|
||||||
--outputs='num_detections,detection_scores,detection_boxes,detection_classes' \
|
|
||||||
--transforms='
|
|
||||||
strip_unused_nodes(type=float, shape="1,300,300,3")
|
|
||||||
remove_nodes(op=Identity, op=CheckNumerics)
|
|
||||||
fold_constants(ignore_errors=true)
|
|
||||||
fold_batch_norms
|
|
||||||
fold_old_batch_norms'
|
|
||||||
```
|
|
||||||
|
49
config/config.yml
Normal file
49
config/config.yml
Normal file
@ -0,0 +1,49 @@
|
|||||||
|
web_port: 5000
|
||||||
|
|
||||||
|
mqtt:
|
||||||
|
host: mqtt.server.com
|
||||||
|
topic_prefix: frigate
|
||||||
|
|
||||||
|
cameras:
|
||||||
|
back:
|
||||||
|
rtsp:
|
||||||
|
user: viewer
|
||||||
|
host: 10.0.10.10
|
||||||
|
port: 554
|
||||||
|
# values that begin with a "$" will be replaced with environment variable
|
||||||
|
password: $RTSP_PASSWORD
|
||||||
|
path: /cam/realmonitor?channel=1&subtype=2
|
||||||
|
regions:
|
||||||
|
- size: 350
|
||||||
|
x_offset: 0
|
||||||
|
y_offset: 300
|
||||||
|
min_person_area: 5000
|
||||||
|
- size: 400
|
||||||
|
x_offset: 350
|
||||||
|
y_offset: 250
|
||||||
|
min_person_area: 2000
|
||||||
|
- size: 400
|
||||||
|
x_offset: 750
|
||||||
|
y_offset: 250
|
||||||
|
min_person_area: 2000
|
||||||
|
back2:
|
||||||
|
rtsp:
|
||||||
|
user: viewer
|
||||||
|
host: 10.0.10.10
|
||||||
|
port: 554
|
||||||
|
# values that begin with a "$" will be replaced with environment variable
|
||||||
|
password: $RTSP_PASSWORD
|
||||||
|
path: /cam/realmonitor?channel=1&subtype=2
|
||||||
|
regions:
|
||||||
|
- size: 350
|
||||||
|
x_offset: 0
|
||||||
|
y_offset: 300
|
||||||
|
min_person_area: 5000
|
||||||
|
- size: 400
|
||||||
|
x_offset: 350
|
||||||
|
y_offset: 250
|
||||||
|
min_person_area: 2000
|
||||||
|
- size: 400
|
||||||
|
x_offset: 750
|
||||||
|
y_offset: 250
|
||||||
|
min_person_area: 2000
|
Binary file not shown.
Before Width: | Height: | Size: 239 KiB |
Binary file not shown.
Before Width: | Height: | Size: 313 KiB |
Binary file not shown.
Before Width: | Height: | Size: 313 KiB |
@ -1,144 +1,27 @@
|
|||||||
import os
|
|
||||||
import cv2
|
import cv2
|
||||||
import imutils
|
|
||||||
import time
|
import time
|
||||||
import datetime
|
import queue
|
||||||
import ctypes
|
import yaml
|
||||||
import logging
|
|
||||||
import multiprocessing as mp
|
|
||||||
import threading
|
|
||||||
import json
|
|
||||||
from contextlib import closing
|
|
||||||
import numpy as np
|
import numpy as np
|
||||||
from object_detection.utils import visualization_utils as vis_util
|
from flask import Flask, Response, make_response
|
||||||
from flask import Flask, Response, make_response, send_file
|
|
||||||
import paho.mqtt.client as mqtt
|
import paho.mqtt.client as mqtt
|
||||||
|
|
||||||
from frigate.util import tonumpyarray
|
from frigate.video import Camera
|
||||||
from frigate.mqtt import MqttMotionPublisher, MqttObjectPublisher
|
from frigate.object_detection import PreppedQueueProcessor
|
||||||
from frigate.objects import ObjectParser, ObjectCleaner, BestPersonFrame
|
|
||||||
from frigate.motion import detect_motion
|
|
||||||
from frigate.video import fetch_frames, FrameTracker
|
|
||||||
from frigate.object_detection import detect_objects
|
|
||||||
|
|
||||||
RTSP_URL = os.getenv('RTSP_URL')
|
with open('/config/config.yml') as f:
|
||||||
|
CONFIG = yaml.safe_load(f)
|
||||||
|
|
||||||
MQTT_HOST = os.getenv('MQTT_HOST')
|
MQTT_HOST = CONFIG['mqtt']['host']
|
||||||
MQTT_USER = os.getenv('MQTT_USER')
|
MQTT_PORT = CONFIG.get('mqtt', {}).get('port', 1883)
|
||||||
MQTT_PASS = os.getenv('MQTT_PASS')
|
MQTT_TOPIC_PREFIX = CONFIG.get('mqtt', {}).get('topic_prefix', 'frigate')
|
||||||
MQTT_TOPIC_PREFIX = os.getenv('MQTT_TOPIC_PREFIX')
|
MQTT_USER = CONFIG.get('mqtt', {}).get('user')
|
||||||
|
MQTT_PASS = CONFIG.get('mqtt', {}).get('password')
|
||||||
|
|
||||||
# REGIONS = "350,0,300,50:400,350,250,50:400,750,250,50"
|
WEB_PORT = CONFIG.get('web_port', 5000)
|
||||||
# REGIONS = "400,350,250,50"
|
DEBUG = (CONFIG.get('debug', '0') == '1')
|
||||||
REGIONS = os.getenv('REGIONS')
|
|
||||||
|
|
||||||
DEBUG = (os.getenv('DEBUG') == '1')
|
|
||||||
|
|
||||||
def main():
|
def main():
|
||||||
DETECTED_OBJECTS = []
|
|
||||||
recent_motion_frames = {}
|
|
||||||
# Parse selected regions
|
|
||||||
regions = []
|
|
||||||
for region_string in REGIONS.split(':'):
|
|
||||||
region_parts = region_string.split(',')
|
|
||||||
region_mask_image = cv2.imread("/config/{}".format(region_parts[5]), cv2.IMREAD_GRAYSCALE)
|
|
||||||
region_mask = np.where(region_mask_image==[0])
|
|
||||||
regions.append({
|
|
||||||
'size': int(region_parts[0]),
|
|
||||||
'x_offset': int(region_parts[1]),
|
|
||||||
'y_offset': int(region_parts[2]),
|
|
||||||
'min_person_area': int(region_parts[3]),
|
|
||||||
'min_object_size': int(region_parts[4]),
|
|
||||||
'mask': region_mask,
|
|
||||||
# Event for motion detection signaling
|
|
||||||
'motion_detected': mp.Event(),
|
|
||||||
# create shared array for storing 10 detected objects
|
|
||||||
# note: this must be a double even though the value you are storing
|
|
||||||
# is a float. otherwise it stops updating the value in shared
|
|
||||||
# memory. probably something to do with the size of the memory block
|
|
||||||
'output_array': mp.Array(ctypes.c_double, 6*10)
|
|
||||||
})
|
|
||||||
# capture a single frame and check the frame shape so the correct array
|
|
||||||
# size can be allocated in memory
|
|
||||||
video = cv2.VideoCapture(RTSP_URL)
|
|
||||||
ret, frame = video.read()
|
|
||||||
if ret:
|
|
||||||
frame_shape = frame.shape
|
|
||||||
else:
|
|
||||||
print("Unable to capture video stream")
|
|
||||||
exit(1)
|
|
||||||
video.release()
|
|
||||||
|
|
||||||
# compute the flattened array length from the array shape
|
|
||||||
flat_array_length = frame_shape[0] * frame_shape[1] * frame_shape[2]
|
|
||||||
# create shared array for storing the full frame image data
|
|
||||||
shared_arr = mp.Array(ctypes.c_uint16, flat_array_length)
|
|
||||||
# create shared value for storing the frame_time
|
|
||||||
shared_frame_time = mp.Value('d', 0.0)
|
|
||||||
# Lock to control access to the frame
|
|
||||||
frame_lock = mp.Lock()
|
|
||||||
# Condition for notifying that a new frame is ready
|
|
||||||
frame_ready = mp.Condition()
|
|
||||||
# Condition for notifying that motion status changed globally
|
|
||||||
motion_changed = mp.Condition()
|
|
||||||
# Condition for notifying that objects were parsed
|
|
||||||
objects_parsed = mp.Condition()
|
|
||||||
# Queue for detected objects
|
|
||||||
object_queue = mp.Queue()
|
|
||||||
|
|
||||||
# shape current frame so it can be treated as an image
|
|
||||||
frame_arr = tonumpyarray(shared_arr).reshape(frame_shape)
|
|
||||||
|
|
||||||
# start the process to capture frames from the RTSP stream and store in a shared array
|
|
||||||
capture_process = mp.Process(target=fetch_frames, args=(shared_arr,
|
|
||||||
shared_frame_time, frame_lock, frame_ready, frame_shape, RTSP_URL))
|
|
||||||
capture_process.daemon = True
|
|
||||||
|
|
||||||
# for each region, start a separate process for motion detection and object detection
|
|
||||||
detection_processes = []
|
|
||||||
motion_processes = []
|
|
||||||
for region in regions:
|
|
||||||
detection_process = mp.Process(target=detect_objects, args=(shared_arr,
|
|
||||||
object_queue,
|
|
||||||
shared_frame_time,
|
|
||||||
frame_lock, frame_ready,
|
|
||||||
region['motion_detected'],
|
|
||||||
frame_shape,
|
|
||||||
region['size'], region['x_offset'], region['y_offset'],
|
|
||||||
region['min_person_area'],
|
|
||||||
DEBUG))
|
|
||||||
detection_process.daemon = True
|
|
||||||
detection_processes.append(detection_process)
|
|
||||||
|
|
||||||
motion_process = mp.Process(target=detect_motion, args=(shared_arr,
|
|
||||||
shared_frame_time,
|
|
||||||
frame_lock, frame_ready,
|
|
||||||
region['motion_detected'],
|
|
||||||
motion_changed,
|
|
||||||
frame_shape,
|
|
||||||
region['size'], region['x_offset'], region['y_offset'],
|
|
||||||
region['min_object_size'], region['mask'],
|
|
||||||
DEBUG))
|
|
||||||
motion_process.daemon = True
|
|
||||||
motion_processes.append(motion_process)
|
|
||||||
|
|
||||||
# start a thread to store recent motion frames for processing
|
|
||||||
frame_tracker = FrameTracker(frame_arr, shared_frame_time, frame_ready, frame_lock,
|
|
||||||
recent_motion_frames, motion_changed, [region['motion_detected'] for region in regions])
|
|
||||||
frame_tracker.start()
|
|
||||||
|
|
||||||
# start a thread to store the highest scoring recent person frame
|
|
||||||
best_person_frame = BestPersonFrame(objects_parsed, recent_motion_frames, DETECTED_OBJECTS,
|
|
||||||
motion_changed, [region['motion_detected'] for region in regions])
|
|
||||||
best_person_frame.start()
|
|
||||||
|
|
||||||
# start a thread to parse objects from the queue
|
|
||||||
object_parser = ObjectParser(object_queue, objects_parsed, DETECTED_OBJECTS)
|
|
||||||
object_parser.start()
|
|
||||||
# start a thread to expire objects from the detected objects list
|
|
||||||
object_cleaner = ObjectCleaner(objects_parsed, DETECTED_OBJECTS)
|
|
||||||
object_cleaner.start()
|
|
||||||
|
|
||||||
# connect to mqtt and setup last will
|
# connect to mqtt and setup last will
|
||||||
def on_connect(client, userdata, flags, rc):
|
def on_connect(client, userdata, flags, rc):
|
||||||
print("On connect called")
|
print("On connect called")
|
||||||
@ -149,99 +32,59 @@ def main():
|
|||||||
client.will_set(MQTT_TOPIC_PREFIX+'/available', payload='offline', qos=1, retain=True)
|
client.will_set(MQTT_TOPIC_PREFIX+'/available', payload='offline', qos=1, retain=True)
|
||||||
if not MQTT_USER is None:
|
if not MQTT_USER is None:
|
||||||
client.username_pw_set(MQTT_USER, password=MQTT_PASS)
|
client.username_pw_set(MQTT_USER, password=MQTT_PASS)
|
||||||
|
client.connect(MQTT_HOST, MQTT_PORT, 60)
|
||||||
client.connect(MQTT_HOST, 1883, 60)
|
|
||||||
client.loop_start()
|
client.loop_start()
|
||||||
|
|
||||||
# start a thread to publish object scores (currently only person)
|
# Queue for prepped frames, max size set to (number of cameras * 5)
|
||||||
mqtt_publisher = MqttObjectPublisher(client, MQTT_TOPIC_PREFIX, objects_parsed, DETECTED_OBJECTS)
|
max_queue_size = len(CONFIG['cameras'].items())*5
|
||||||
mqtt_publisher.start()
|
prepped_frame_queue = queue.Queue(max_queue_size)
|
||||||
|
|
||||||
# start thread to publish motion status
|
cameras = {}
|
||||||
mqtt_motion_publisher = MqttMotionPublisher(client, MQTT_TOPIC_PREFIX, motion_changed,
|
for name, config in CONFIG['cameras'].items():
|
||||||
[region['motion_detected'] for region in regions])
|
cameras[name] = Camera(name, config, prepped_frame_queue, client, MQTT_TOPIC_PREFIX)
|
||||||
mqtt_motion_publisher.start()
|
|
||||||
|
|
||||||
# start the process of capturing frames
|
prepped_queue_processor = PreppedQueueProcessor(
|
||||||
capture_process.start()
|
cameras,
|
||||||
print("capture_process pid ", capture_process.pid)
|
prepped_frame_queue
|
||||||
|
)
|
||||||
|
prepped_queue_processor.start()
|
||||||
|
|
||||||
# start the object detection processes
|
for name, camera in cameras.items():
|
||||||
for detection_process in detection_processes:
|
camera.start()
|
||||||
detection_process.start()
|
print("Capture process for {}: {}".format(name, camera.get_capture_pid()))
|
||||||
print("detection_process pid ", detection_process.pid)
|
|
||||||
|
|
||||||
# start the motion detection processes
|
|
||||||
for motion_process in motion_processes:
|
|
||||||
motion_process.start()
|
|
||||||
print("motion_process pid ", motion_process.pid)
|
|
||||||
|
|
||||||
# create a flask app that encodes frames a mjpeg on demand
|
# create a flask app that encodes frames a mjpeg on demand
|
||||||
app = Flask(__name__)
|
app = Flask(__name__)
|
||||||
|
|
||||||
@app.route('/best_person.jpg')
|
@app.route('/<camera_name>/best_person.jpg')
|
||||||
def best_person():
|
def best_person(camera_name):
|
||||||
frame = np.zeros(frame_shape, np.uint8) if best_person_frame.best_frame is None else best_person_frame.best_frame
|
best_person_frame = cameras[camera_name].get_best_person()
|
||||||
ret, jpg = cv2.imencode('.jpg', frame)
|
if best_person_frame is None:
|
||||||
|
best_person_frame = np.zeros((720,1280,3), np.uint8)
|
||||||
|
ret, jpg = cv2.imencode('.jpg', best_person_frame)
|
||||||
response = make_response(jpg.tobytes())
|
response = make_response(jpg.tobytes())
|
||||||
response.headers['Content-Type'] = 'image/jpg'
|
response.headers['Content-Type'] = 'image/jpg'
|
||||||
return response
|
return response
|
||||||
|
|
||||||
@app.route('/')
|
@app.route('/<camera_name>')
|
||||||
def index():
|
def mjpeg_feed(camera_name):
|
||||||
# return a multipart response
|
# return a multipart response
|
||||||
return Response(imagestream(),
|
return Response(imagestream(camera_name),
|
||||||
mimetype='multipart/x-mixed-replace; boundary=frame')
|
mimetype='multipart/x-mixed-replace; boundary=frame')
|
||||||
def imagestream():
|
|
||||||
|
def imagestream(camera_name):
|
||||||
while True:
|
while True:
|
||||||
# max out at 5 FPS
|
# max out at 5 FPS
|
||||||
time.sleep(0.2)
|
time.sleep(0.2)
|
||||||
# make a copy of the current detected objects
|
frame = cameras[camera_name].get_current_frame_with_objects()
|
||||||
detected_objects = DETECTED_OBJECTS.copy()
|
|
||||||
# lock and make a copy of the current frame
|
|
||||||
with frame_lock:
|
|
||||||
frame = frame_arr.copy()
|
|
||||||
# convert to RGB for drawing
|
|
||||||
frame = cv2.cvtColor(frame, cv2.COLOR_BGR2RGB)
|
|
||||||
# draw the bounding boxes on the screen
|
|
||||||
for obj in detected_objects:
|
|
||||||
vis_util.draw_bounding_box_on_image_array(frame,
|
|
||||||
obj['ymin'],
|
|
||||||
obj['xmin'],
|
|
||||||
obj['ymax'],
|
|
||||||
obj['xmax'],
|
|
||||||
color='red',
|
|
||||||
thickness=2,
|
|
||||||
display_str_list=["{}: {}%".format(obj['name'],int(obj['score']*100))],
|
|
||||||
use_normalized_coordinates=False)
|
|
||||||
|
|
||||||
for region in regions:
|
|
||||||
color = (255,255,255)
|
|
||||||
if region['motion_detected'].is_set():
|
|
||||||
color = (0,255,0)
|
|
||||||
cv2.rectangle(frame, (region['x_offset'], region['y_offset']),
|
|
||||||
(region['x_offset']+region['size'], region['y_offset']+region['size']),
|
|
||||||
color, 2)
|
|
||||||
|
|
||||||
# convert back to BGR
|
|
||||||
frame = cv2.cvtColor(frame, cv2.COLOR_RGB2BGR)
|
|
||||||
# encode the image into a jpg
|
# encode the image into a jpg
|
||||||
ret, jpg = cv2.imencode('.jpg', frame)
|
ret, jpg = cv2.imencode('.jpg', frame)
|
||||||
yield (b'--frame\r\n'
|
yield (b'--frame\r\n'
|
||||||
b'Content-Type: image/jpeg\r\n\r\n' + jpg.tobytes() + b'\r\n\r\n')
|
b'Content-Type: image/jpeg\r\n\r\n' + jpg.tobytes() + b'\r\n\r\n')
|
||||||
|
|
||||||
app.run(host='0.0.0.0', debug=False)
|
app.run(host='0.0.0.0', port=WEB_PORT, debug=False)
|
||||||
|
|
||||||
capture_process.join()
|
camera.join()
|
||||||
for detection_process in detection_processes:
|
|
||||||
detection_process.join()
|
|
||||||
for motion_process in motion_processes:
|
|
||||||
motion_process.join()
|
|
||||||
frame_tracker.join()
|
|
||||||
best_person_frame.join()
|
|
||||||
object_parser.join()
|
|
||||||
object_cleaner.join()
|
|
||||||
mqtt_publisher.join()
|
|
||||||
|
|
||||||
if __name__ == '__main__':
|
if __name__ == '__main__':
|
||||||
main()
|
main()
|
BIN
diagram.png
BIN
diagram.png
Binary file not shown.
Before Width: | Height: | Size: 308 KiB After Width: | Height: | Size: 283 KiB |
@ -1,109 +0,0 @@
|
|||||||
import datetime
|
|
||||||
import numpy as np
|
|
||||||
import cv2
|
|
||||||
import imutils
|
|
||||||
from . util import tonumpyarray
|
|
||||||
|
|
||||||
# do the actual motion detection
|
|
||||||
def detect_motion(shared_arr, shared_frame_time, frame_lock, frame_ready, motion_detected, motion_changed,
|
|
||||||
frame_shape, region_size, region_x_offset, region_y_offset, min_motion_area, mask, debug):
|
|
||||||
# shape shared input array into frame for processing
|
|
||||||
arr = tonumpyarray(shared_arr).reshape(frame_shape)
|
|
||||||
|
|
||||||
avg_frame = None
|
|
||||||
avg_delta = None
|
|
||||||
frame_time = 0.0
|
|
||||||
motion_frames = 0
|
|
||||||
while True:
|
|
||||||
now = datetime.datetime.now().timestamp()
|
|
||||||
|
|
||||||
with frame_ready:
|
|
||||||
# if there isnt a frame ready for processing or it is old, wait for a signal
|
|
||||||
if shared_frame_time.value == frame_time or (now - shared_frame_time.value) > 0.5:
|
|
||||||
frame_ready.wait()
|
|
||||||
|
|
||||||
# lock and make a copy of the cropped frame
|
|
||||||
with frame_lock:
|
|
||||||
cropped_frame = arr[region_y_offset:region_y_offset+region_size, region_x_offset:region_x_offset+region_size].copy().astype('uint8')
|
|
||||||
frame_time = shared_frame_time.value
|
|
||||||
|
|
||||||
# convert to grayscale
|
|
||||||
gray = cv2.cvtColor(cropped_frame, cv2.COLOR_BGR2GRAY)
|
|
||||||
|
|
||||||
# apply image mask to remove areas from motion detection
|
|
||||||
gray[mask] = [255]
|
|
||||||
|
|
||||||
# apply gaussian blur
|
|
||||||
gray = cv2.GaussianBlur(gray, (21, 21), 0)
|
|
||||||
|
|
||||||
if avg_frame is None:
|
|
||||||
avg_frame = gray.copy().astype("float")
|
|
||||||
continue
|
|
||||||
|
|
||||||
# look at the delta from the avg_frame
|
|
||||||
frameDelta = cv2.absdiff(gray, cv2.convertScaleAbs(avg_frame))
|
|
||||||
|
|
||||||
if avg_delta is None:
|
|
||||||
avg_delta = frameDelta.copy().astype("float")
|
|
||||||
|
|
||||||
# compute the average delta over the past few frames
|
|
||||||
# the alpha value can be modified to configure how sensitive the motion detection is.
|
|
||||||
# higher values mean the current frame impacts the delta a lot, and a single raindrop may
|
|
||||||
# register as motion, too low and a fast moving person wont be detected as motion
|
|
||||||
# this also assumes that a person is in the same location across more than a single frame
|
|
||||||
cv2.accumulateWeighted(frameDelta, avg_delta, 0.2)
|
|
||||||
|
|
||||||
# compute the threshold image for the current frame
|
|
||||||
current_thresh = cv2.threshold(frameDelta, 25, 255, cv2.THRESH_BINARY)[1]
|
|
||||||
|
|
||||||
# black out everything in the avg_delta where there isnt motion in the current frame
|
|
||||||
avg_delta_image = cv2.convertScaleAbs(avg_delta)
|
|
||||||
avg_delta_image[np.where(current_thresh==[0])] = [0]
|
|
||||||
|
|
||||||
# then look for deltas above the threshold, but only in areas where there is a delta
|
|
||||||
# in the current frame. this prevents deltas from previous frames from being included
|
|
||||||
thresh = cv2.threshold(avg_delta_image, 25, 255, cv2.THRESH_BINARY)[1]
|
|
||||||
|
|
||||||
# dilate the thresholded image to fill in holes, then find contours
|
|
||||||
# on thresholded image
|
|
||||||
thresh = cv2.dilate(thresh, None, iterations=2)
|
|
||||||
cnts = cv2.findContours(thresh.copy(), cv2.RETR_EXTERNAL, cv2.CHAIN_APPROX_SIMPLE)
|
|
||||||
cnts = imutils.grab_contours(cnts)
|
|
||||||
|
|
||||||
motion_found = False
|
|
||||||
|
|
||||||
# loop over the contours
|
|
||||||
for c in cnts:
|
|
||||||
# if the contour is big enough, count it as motion
|
|
||||||
contour_area = cv2.contourArea(c)
|
|
||||||
if contour_area > min_motion_area:
|
|
||||||
motion_found = True
|
|
||||||
if debug:
|
|
||||||
cv2.drawContours(cropped_frame, [c], -1, (0, 255, 0), 2)
|
|
||||||
x, y, w, h = cv2.boundingRect(c)
|
|
||||||
cv2.putText(cropped_frame, str(contour_area), (x, y),
|
|
||||||
cv2.FONT_HERSHEY_SIMPLEX, 0.5, (0, 100, 0), 2)
|
|
||||||
else:
|
|
||||||
break
|
|
||||||
|
|
||||||
if motion_found:
|
|
||||||
motion_frames += 1
|
|
||||||
# if there have been enough consecutive motion frames, report motion
|
|
||||||
if motion_frames >= 3:
|
|
||||||
# only average in the current frame if the difference persists for at least 3 frames
|
|
||||||
cv2.accumulateWeighted(gray, avg_frame, 0.01)
|
|
||||||
motion_detected.set()
|
|
||||||
with motion_changed:
|
|
||||||
motion_changed.notify_all()
|
|
||||||
else:
|
|
||||||
# when no motion, just keep averaging the frames together
|
|
||||||
cv2.accumulateWeighted(gray, avg_frame, 0.01)
|
|
||||||
motion_frames = 0
|
|
||||||
if motion_detected.is_set():
|
|
||||||
motion_detected.clear()
|
|
||||||
with motion_changed:
|
|
||||||
motion_changed.notify_all()
|
|
||||||
|
|
||||||
if debug and motion_frames == 3:
|
|
||||||
cv2.imwrite("/lab/debug/motion-{}-{}-{}.jpg".format(region_x_offset, region_y_offset, datetime.datetime.now().timestamp()), cropped_frame)
|
|
||||||
cv2.imwrite("/lab/debug/avg_delta-{}-{}-{}.jpg".format(region_x_offset, region_y_offset, datetime.datetime.now().timestamp()), avg_delta_image)
|
|
@ -1,29 +1,6 @@
|
|||||||
import json
|
import json
|
||||||
import threading
|
import threading
|
||||||
|
|
||||||
class MqttMotionPublisher(threading.Thread):
|
|
||||||
def __init__(self, client, topic_prefix, motion_changed, motion_flags):
|
|
||||||
threading.Thread.__init__(self)
|
|
||||||
self.client = client
|
|
||||||
self.topic_prefix = topic_prefix
|
|
||||||
self.motion_changed = motion_changed
|
|
||||||
self.motion_flags = motion_flags
|
|
||||||
|
|
||||||
def run(self):
|
|
||||||
last_sent_motion = ""
|
|
||||||
while True:
|
|
||||||
with self.motion_changed:
|
|
||||||
self.motion_changed.wait()
|
|
||||||
|
|
||||||
# send message for motion
|
|
||||||
motion_status = 'OFF'
|
|
||||||
if any(obj.is_set() for obj in self.motion_flags):
|
|
||||||
motion_status = 'ON'
|
|
||||||
|
|
||||||
if last_sent_motion != motion_status:
|
|
||||||
last_sent_motion = motion_status
|
|
||||||
self.client.publish(self.topic_prefix+'/motion', motion_status, retain=False)
|
|
||||||
|
|
||||||
class MqttObjectPublisher(threading.Thread):
|
class MqttObjectPublisher(threading.Thread):
|
||||||
def __init__(self, client, topic_prefix, objects_parsed, detected_objects):
|
def __init__(self, client, topic_prefix, objects_parsed, detected_objects):
|
||||||
threading.Thread.__init__(self)
|
threading.Thread.__init__(self)
|
||||||
@ -43,11 +20,11 @@ class MqttObjectPublisher(threading.Thread):
|
|||||||
with self.objects_parsed:
|
with self.objects_parsed:
|
||||||
self.objects_parsed.wait()
|
self.objects_parsed.wait()
|
||||||
|
|
||||||
# add all the person scores in detected objects and
|
# add all the person scores in detected objects
|
||||||
# average over past 1 seconds (5fps)
|
|
||||||
detected_objects = self._detected_objects.copy()
|
detected_objects = self._detected_objects.copy()
|
||||||
avg_person_score = sum([obj['score'] for obj in detected_objects if obj['name'] == 'person'])/5
|
person_score = sum([obj['score'] for obj in detected_objects if obj['name'] == 'person'])
|
||||||
payload['person'] = int(avg_person_score*100)
|
# if the person score is more than 100, set person to ON
|
||||||
|
payload['person'] = 'ON' if int(person_score*100) > 100 else 'OFF'
|
||||||
|
|
||||||
# send message for objects if different
|
# send message for objects if different
|
||||||
new_payload = json.dumps(payload, sort_keys=True)
|
new_payload = json.dumps(payload, sort_keys=True)
|
||||||
|
@ -1,114 +1,110 @@
|
|||||||
import datetime
|
import datetime
|
||||||
|
import time
|
||||||
import cv2
|
import cv2
|
||||||
|
import threading
|
||||||
import numpy as np
|
import numpy as np
|
||||||
import tensorflow as tf
|
from edgetpu.detection.engine import DetectionEngine
|
||||||
from object_detection.utils import label_map_util
|
|
||||||
from object_detection.utils import visualization_utils as vis_util
|
|
||||||
from . util import tonumpyarray
|
from . util import tonumpyarray
|
||||||
|
|
||||||
# TODO: make dynamic?
|
|
||||||
NUM_CLASSES = 90
|
|
||||||
# Path to frozen detection graph. This is the actual model that is used for the object detection.
|
# Path to frozen detection graph. This is the actual model that is used for the object detection.
|
||||||
PATH_TO_CKPT = '/frozen_inference_graph.pb'
|
PATH_TO_CKPT = '/frozen_inference_graph.pb'
|
||||||
# List of the strings that is used to add correct label for each box.
|
# List of the strings that is used to add correct label for each box.
|
||||||
PATH_TO_LABELS = '/label_map.pbtext'
|
PATH_TO_LABELS = '/label_map.pbtext'
|
||||||
|
|
||||||
# Loading label map
|
# Function to read labels from text files.
|
||||||
label_map = label_map_util.load_labelmap(PATH_TO_LABELS)
|
def ReadLabelFile(file_path):
|
||||||
categories = label_map_util.convert_label_map_to_categories(label_map, max_num_classes=NUM_CLASSES,
|
with open(file_path, 'r') as f:
|
||||||
use_display_name=True)
|
lines = f.readlines()
|
||||||
category_index = label_map_util.create_category_index(categories)
|
ret = {}
|
||||||
|
for line in lines:
|
||||||
|
pair = line.strip().split(maxsplit=1)
|
||||||
|
ret[int(pair[0])] = pair[1].strip()
|
||||||
|
return ret
|
||||||
|
|
||||||
# do the actual object detection
|
class PreppedQueueProcessor(threading.Thread):
|
||||||
def tf_detect_objects(cropped_frame, sess, detection_graph, region_size, region_x_offset, region_y_offset, debug):
|
def __init__(self, cameras, prepped_frame_queue):
|
||||||
# Expand dimensions since the model expects images to have shape: [1, None, None, 3]
|
|
||||||
image_np_expanded = np.expand_dims(cropped_frame, axis=0)
|
|
||||||
image_tensor = detection_graph.get_tensor_by_name('image_tensor:0')
|
|
||||||
|
|
||||||
# Each box represents a part of the image where a particular object was detected.
|
threading.Thread.__init__(self)
|
||||||
boxes = detection_graph.get_tensor_by_name('detection_boxes:0')
|
self.cameras = cameras
|
||||||
|
self.prepped_frame_queue = prepped_frame_queue
|
||||||
|
|
||||||
# Each score represent how level of confidence for each of the objects.
|
# Load the edgetpu engine and labels
|
||||||
# Score is shown on the result image, together with the class label.
|
self.engine = DetectionEngine(PATH_TO_CKPT)
|
||||||
scores = detection_graph.get_tensor_by_name('detection_scores:0')
|
self.labels = ReadLabelFile(PATH_TO_LABELS)
|
||||||
classes = detection_graph.get_tensor_by_name('detection_classes:0')
|
|
||||||
num_detections = detection_graph.get_tensor_by_name('num_detections:0')
|
def run(self):
|
||||||
|
# process queue...
|
||||||
|
while True:
|
||||||
|
frame = self.prepped_frame_queue.get()
|
||||||
|
|
||||||
# Actual detection.
|
# Actual detection.
|
||||||
(boxes, scores, classes, num_detections) = sess.run(
|
objects = self.engine.DetectWithInputTensor(frame['frame'], threshold=0.5, top_k=3)
|
||||||
[boxes, scores, classes, num_detections],
|
# parse and pass detected objects back to the camera
|
||||||
feed_dict={image_tensor: image_np_expanded})
|
parsed_objects = []
|
||||||
|
for obj in objects:
|
||||||
if debug:
|
box = obj.bounding_box.flatten().tolist()
|
||||||
if len([value for index,value in enumerate(classes[0]) if str(category_index.get(value).get('name')) == 'person' and scores[0,index] > 0.5]) > 0:
|
parsed_objects.append({
|
||||||
vis_util.visualize_boxes_and_labels_on_image_array(
|
'frame_time': frame['frame_time'],
|
||||||
cropped_frame,
|
'name': str(self.labels[obj.label_id]),
|
||||||
np.squeeze(boxes),
|
'score': float(obj.score),
|
||||||
np.squeeze(classes).astype(np.int32),
|
'xmin': int((box[0] * frame['region_size']) + frame['region_x_offset']),
|
||||||
np.squeeze(scores),
|
'ymin': int((box[1] * frame['region_size']) + frame['region_y_offset']),
|
||||||
category_index,
|
'xmax': int((box[2] * frame['region_size']) + frame['region_x_offset']),
|
||||||
use_normalized_coordinates=True,
|
'ymax': int((box[3] * frame['region_size']) + frame['region_y_offset'])
|
||||||
line_thickness=4)
|
|
||||||
cv2.imwrite("/lab/debug/obj-{}-{}-{}.jpg".format(region_x_offset, region_y_offset, datetime.datetime.now().timestamp()), cropped_frame)
|
|
||||||
|
|
||||||
|
|
||||||
# build an array of detected objects
|
|
||||||
objects = []
|
|
||||||
for index, value in enumerate(classes[0]):
|
|
||||||
score = scores[0, index]
|
|
||||||
if score > 0.5:
|
|
||||||
box = boxes[0, index].tolist()
|
|
||||||
objects.append({
|
|
||||||
'name': str(category_index.get(value).get('name')),
|
|
||||||
'score': float(score),
|
|
||||||
'ymin': int((box[0] * region_size) + region_y_offset),
|
|
||||||
'xmin': int((box[1] * region_size) + region_x_offset),
|
|
||||||
'ymax': int((box[2] * region_size) + region_y_offset),
|
|
||||||
'xmax': int((box[3] * region_size) + region_x_offset)
|
|
||||||
})
|
})
|
||||||
|
self.cameras[frame['camera_name']].add_objects(parsed_objects)
|
||||||
|
|
||||||
return objects
|
|
||||||
|
|
||||||
def detect_objects(shared_arr, object_queue, shared_frame_time, frame_lock, frame_ready,
|
# should this be a region class?
|
||||||
motion_detected, frame_shape, region_size, region_x_offset, region_y_offset,
|
class FramePrepper(threading.Thread):
|
||||||
min_person_area, debug):
|
def __init__(self, camera_name, shared_frame, frame_time, frame_ready,
|
||||||
# shape shared input array into frame for processing
|
frame_lock,
|
||||||
arr = tonumpyarray(shared_arr).reshape(frame_shape)
|
region_size, region_x_offset, region_y_offset,
|
||||||
|
prepped_frame_queue):
|
||||||
|
|
||||||
# Load a (frozen) Tensorflow model into memory before the processing loop
|
threading.Thread.__init__(self)
|
||||||
detection_graph = tf.Graph()
|
self.camera_name = camera_name
|
||||||
with detection_graph.as_default():
|
self.shared_frame = shared_frame
|
||||||
od_graph_def = tf.GraphDef()
|
self.frame_time = frame_time
|
||||||
with tf.gfile.GFile(PATH_TO_CKPT, 'rb') as fid:
|
self.frame_ready = frame_ready
|
||||||
serialized_graph = fid.read()
|
self.frame_lock = frame_lock
|
||||||
od_graph_def.ParseFromString(serialized_graph)
|
self.region_size = region_size
|
||||||
tf.import_graph_def(od_graph_def, name='')
|
self.region_x_offset = region_x_offset
|
||||||
sess = tf.Session(graph=detection_graph)
|
self.region_y_offset = region_y_offset
|
||||||
|
self.prepped_frame_queue = prepped_frame_queue
|
||||||
|
|
||||||
|
def run(self):
|
||||||
frame_time = 0.0
|
frame_time = 0.0
|
||||||
while True:
|
while True:
|
||||||
now = datetime.datetime.now().timestamp()
|
now = datetime.datetime.now().timestamp()
|
||||||
|
|
||||||
# wait until motion is detected
|
with self.frame_ready:
|
||||||
motion_detected.wait()
|
|
||||||
|
|
||||||
with frame_ready:
|
|
||||||
# if there isnt a frame ready for processing or it is old, wait for a new frame
|
# if there isnt a frame ready for processing or it is old, wait for a new frame
|
||||||
if shared_frame_time.value == frame_time or (now - shared_frame_time.value) > 0.5:
|
if self.frame_time.value == frame_time or (now - self.frame_time.value) > 0.5:
|
||||||
frame_ready.wait()
|
self.frame_ready.wait()
|
||||||
|
|
||||||
# make a copy of the cropped frame
|
# make a copy of the cropped frame
|
||||||
with frame_lock:
|
with self.frame_lock:
|
||||||
cropped_frame = arr[region_y_offset:region_y_offset+region_size, region_x_offset:region_x_offset+region_size].copy()
|
cropped_frame = self.shared_frame[self.region_y_offset:self.region_y_offset+self.region_size, self.region_x_offset:self.region_x_offset+self.region_size].copy()
|
||||||
frame_time = shared_frame_time.value
|
frame_time = self.frame_time.value
|
||||||
|
|
||||||
# convert to RGB
|
# convert to RGB
|
||||||
cropped_frame_rgb = cv2.cvtColor(cropped_frame, cv2.COLOR_BGR2RGB)
|
cropped_frame_rgb = cv2.cvtColor(cropped_frame, cv2.COLOR_BGR2RGB)
|
||||||
# do the object detection
|
# Resize to 300x300 if needed
|
||||||
objects = tf_detect_objects(cropped_frame_rgb, sess, detection_graph, region_size, region_x_offset, region_y_offset, debug)
|
if cropped_frame_rgb.shape != (300, 300, 3):
|
||||||
for obj in objects:
|
cropped_frame_rgb = cv2.resize(cropped_frame_rgb, dsize=(300, 300), interpolation=cv2.INTER_LINEAR)
|
||||||
# ignore persons below the size threshold
|
# Expand dimensions since the model expects images to have shape: [1, 300, 300, 3]
|
||||||
if obj['name'] == 'person' and (obj['xmax']-obj['xmin'])*(obj['ymax']-obj['ymin']) < min_person_area:
|
frame_expanded = np.expand_dims(cropped_frame_rgb, axis=0)
|
||||||
continue
|
|
||||||
obj['frame_time'] = frame_time
|
# add the frame to the queue
|
||||||
object_queue.put(obj)
|
if not self.prepped_frame_queue.full():
|
||||||
|
self.prepped_frame_queue.put({
|
||||||
|
'camera_name': self.camera_name,
|
||||||
|
'frame_time': frame_time,
|
||||||
|
'frame': frame_expanded.flatten().copy(),
|
||||||
|
'region_size': self.region_size,
|
||||||
|
'region_x_offset': self.region_x_offset,
|
||||||
|
'region_y_offset': self.region_y_offset
|
||||||
|
})
|
||||||
|
else:
|
||||||
|
print("queue full. moving on")
|
||||||
|
@ -3,21 +3,6 @@ import datetime
|
|||||||
import threading
|
import threading
|
||||||
import cv2
|
import cv2
|
||||||
from object_detection.utils import visualization_utils as vis_util
|
from object_detection.utils import visualization_utils as vis_util
|
||||||
class ObjectParser(threading.Thread):
|
|
||||||
def __init__(self, object_queue, objects_parsed, detected_objects):
|
|
||||||
threading.Thread.__init__(self)
|
|
||||||
self._object_queue = object_queue
|
|
||||||
self._objects_parsed = objects_parsed
|
|
||||||
self._detected_objects = detected_objects
|
|
||||||
|
|
||||||
def run(self):
|
|
||||||
while True:
|
|
||||||
obj = self._object_queue.get()
|
|
||||||
self._detected_objects.append(obj)
|
|
||||||
|
|
||||||
# notify that objects were parsed
|
|
||||||
with self._objects_parsed:
|
|
||||||
self._objects_parsed.notify_all()
|
|
||||||
|
|
||||||
class ObjectCleaner(threading.Thread):
|
class ObjectCleaner(threading.Thread):
|
||||||
def __init__(self, objects_parsed, detected_objects):
|
def __init__(self, objects_parsed, detected_objects):
|
||||||
@ -28,14 +13,18 @@ class ObjectCleaner(threading.Thread):
|
|||||||
def run(self):
|
def run(self):
|
||||||
while True:
|
while True:
|
||||||
|
|
||||||
|
# wait a bit before checking for expired frames
|
||||||
|
time.sleep(0.2)
|
||||||
|
|
||||||
# expire the objects that are more than 1 second old
|
# expire the objects that are more than 1 second old
|
||||||
now = datetime.datetime.now().timestamp()
|
now = datetime.datetime.now().timestamp()
|
||||||
# look for the first object found within the last second
|
# look for the first object found within the last second
|
||||||
# (newest objects are appended to the end)
|
# (newest objects are appended to the end)
|
||||||
detected_objects = self._detected_objects.copy()
|
detected_objects = self._detected_objects.copy()
|
||||||
|
|
||||||
num_to_delete = 0
|
num_to_delete = 0
|
||||||
for obj in detected_objects:
|
for obj in detected_objects:
|
||||||
if now-obj['frame_time']<1:
|
if now-obj['frame_time']<2:
|
||||||
break
|
break
|
||||||
num_to_delete += 1
|
num_to_delete += 1
|
||||||
if num_to_delete > 0:
|
if num_to_delete > 0:
|
||||||
@ -45,30 +34,21 @@ class ObjectCleaner(threading.Thread):
|
|||||||
with self._objects_parsed:
|
with self._objects_parsed:
|
||||||
self._objects_parsed.notify_all()
|
self._objects_parsed.notify_all()
|
||||||
|
|
||||||
# wait a bit before checking for more expired frames
|
|
||||||
time.sleep(0.2)
|
|
||||||
|
|
||||||
# Maintains the frame and person with the highest score from the most recent
|
# Maintains the frame and person with the highest score from the most recent
|
||||||
# motion event
|
# motion event
|
||||||
class BestPersonFrame(threading.Thread):
|
class BestPersonFrame(threading.Thread):
|
||||||
def __init__(self, objects_parsed, recent_frames, detected_objects, motion_changed, motion_regions):
|
def __init__(self, objects_parsed, recent_frames, detected_objects):
|
||||||
threading.Thread.__init__(self)
|
threading.Thread.__init__(self)
|
||||||
self.objects_parsed = objects_parsed
|
self.objects_parsed = objects_parsed
|
||||||
self.recent_frames = recent_frames
|
self.recent_frames = recent_frames
|
||||||
self.detected_objects = detected_objects
|
self.detected_objects = detected_objects
|
||||||
self.motion_changed = motion_changed
|
|
||||||
self.motion_regions = motion_regions
|
|
||||||
self.best_person = None
|
self.best_person = None
|
||||||
self.best_frame = None
|
self.best_frame = None
|
||||||
|
|
||||||
def run(self):
|
def run(self):
|
||||||
motion_start = 0.0
|
|
||||||
motion_end = 0.0
|
|
||||||
|
|
||||||
while True:
|
while True:
|
||||||
|
|
||||||
# while there is motion
|
|
||||||
while len([r for r in self.motion_regions if r.is_set()]) > 0:
|
|
||||||
# wait until objects have been parsed
|
# wait until objects have been parsed
|
||||||
with self.objects_parsed:
|
with self.objects_parsed:
|
||||||
self.objects_parsed.wait()
|
self.objects_parsed.wait()
|
||||||
@ -76,8 +56,6 @@ class BestPersonFrame(threading.Thread):
|
|||||||
# make a copy of detected objects
|
# make a copy of detected objects
|
||||||
detected_objects = self.detected_objects.copy()
|
detected_objects = self.detected_objects.copy()
|
||||||
detected_people = [obj for obj in detected_objects if obj['name'] == 'person']
|
detected_people = [obj for obj in detected_objects if obj['name'] == 'person']
|
||||||
# make a copy of the recent frames
|
|
||||||
recent_frames = self.recent_frames.copy()
|
|
||||||
|
|
||||||
# get the highest scoring person
|
# get the highest scoring person
|
||||||
new_best_person = max(detected_people, key=lambda x:x['score'], default=self.best_person)
|
new_best_person = max(detected_people, key=lambda x:x['score'], default=self.best_person)
|
||||||
@ -97,6 +75,9 @@ class BestPersonFrame(threading.Thread):
|
|||||||
if new_best_person['score'] > self.best_person['score'] or (now - self.best_person['frame_time']) > 60:
|
if new_best_person['score'] > self.best_person['score'] or (now - self.best_person['frame_time']) > 60:
|
||||||
self.best_person = new_best_person
|
self.best_person = new_best_person
|
||||||
|
|
||||||
|
# make a copy of the recent frames
|
||||||
|
recent_frames = self.recent_frames.copy()
|
||||||
|
|
||||||
if not self.best_person is None and self.best_person['frame_time'] in recent_frames:
|
if not self.best_person is None and self.best_person['frame_time'] in recent_frames:
|
||||||
best_frame = recent_frames[self.best_person['frame_time']]
|
best_frame = recent_frames[self.best_person['frame_time']]
|
||||||
best_frame = cv2.cvtColor(best_frame, cv2.COLOR_BGR2RGB)
|
best_frame = cv2.cvtColor(best_frame, cv2.COLOR_BGR2RGB)
|
||||||
@ -113,11 +94,3 @@ class BestPersonFrame(threading.Thread):
|
|||||||
|
|
||||||
# convert back to BGR
|
# convert back to BGR
|
||||||
self.best_frame = cv2.cvtColor(best_frame, cv2.COLOR_RGB2BGR)
|
self.best_frame = cv2.cvtColor(best_frame, cv2.COLOR_RGB2BGR)
|
||||||
|
|
||||||
motion_end = datetime.datetime.now().timestamp()
|
|
||||||
|
|
||||||
# wait for the global motion flag to change
|
|
||||||
with self.motion_changed:
|
|
||||||
self.motion_changed.wait()
|
|
||||||
|
|
||||||
motion_start = datetime.datetime.now().timestamp()
|
|
@ -2,4 +2,4 @@ import numpy as np
|
|||||||
|
|
||||||
# convert shared memory array into numpy array
|
# convert shared memory array into numpy array
|
||||||
def tonumpyarray(mp_arr):
|
def tonumpyarray(mp_arr):
|
||||||
return np.frombuffer(mp_arr.get_obj(), dtype=np.uint16)
|
return np.frombuffer(mp_arr.get_obj(), dtype=np.uint8)
|
179
frigate/video.py
179
frigate/video.py
@ -1,11 +1,17 @@
|
|||||||
|
import os
|
||||||
import time
|
import time
|
||||||
import datetime
|
import datetime
|
||||||
import cv2
|
import cv2
|
||||||
import threading
|
import threading
|
||||||
|
import ctypes
|
||||||
|
import multiprocessing as mp
|
||||||
|
from object_detection.utils import visualization_utils as vis_util
|
||||||
from . util import tonumpyarray
|
from . util import tonumpyarray
|
||||||
|
from . object_detection import FramePrepper
|
||||||
|
from . objects import ObjectCleaner, BestPersonFrame
|
||||||
|
from . mqtt import MqttObjectPublisher
|
||||||
|
|
||||||
# fetch the frames as fast a possible, only decoding the frames when the
|
# fetch the frames as fast a possible and store current frame in a shared memory array
|
||||||
# detection_process has consumed the current frame
|
|
||||||
def fetch_frames(shared_arr, shared_frame_time, frame_lock, frame_ready, frame_shape, rtsp_url):
|
def fetch_frames(shared_arr, shared_frame_time, frame_lock, frame_ready, frame_shape, rtsp_url):
|
||||||
# convert shared memory array into numpy and shape into image array
|
# convert shared memory array into numpy and shape into image array
|
||||||
arr = tonumpyarray(shared_arr).reshape(frame_shape)
|
arr = tonumpyarray(shared_arr).reshape(frame_shape)
|
||||||
@ -54,21 +60,17 @@ def fetch_frames(shared_arr, shared_frame_time, frame_lock, frame_ready, frame_s
|
|||||||
|
|
||||||
# Stores 2 seconds worth of frames when motion is detected so they can be used for other threads
|
# Stores 2 seconds worth of frames when motion is detected so they can be used for other threads
|
||||||
class FrameTracker(threading.Thread):
|
class FrameTracker(threading.Thread):
|
||||||
def __init__(self, shared_frame, frame_time, frame_ready, frame_lock, recent_frames, motion_changed, motion_regions):
|
def __init__(self, shared_frame, frame_time, frame_ready, frame_lock, recent_frames):
|
||||||
threading.Thread.__init__(self)
|
threading.Thread.__init__(self)
|
||||||
self.shared_frame = shared_frame
|
self.shared_frame = shared_frame
|
||||||
self.frame_time = frame_time
|
self.frame_time = frame_time
|
||||||
self.frame_ready = frame_ready
|
self.frame_ready = frame_ready
|
||||||
self.frame_lock = frame_lock
|
self.frame_lock = frame_lock
|
||||||
self.recent_frames = recent_frames
|
self.recent_frames = recent_frames
|
||||||
self.motion_changed = motion_changed
|
|
||||||
self.motion_regions = motion_regions
|
|
||||||
|
|
||||||
def run(self):
|
def run(self):
|
||||||
frame_time = 0.0
|
frame_time = 0.0
|
||||||
while True:
|
while True:
|
||||||
# while there is motion
|
|
||||||
while len([r for r in self.motion_regions if r.is_set()]) > 0:
|
|
||||||
now = datetime.datetime.now().timestamp()
|
now = datetime.datetime.now().timestamp()
|
||||||
# wait for a frame
|
# wait for a frame
|
||||||
with self.frame_ready:
|
with self.frame_ready:
|
||||||
@ -78,7 +80,7 @@ class FrameTracker(threading.Thread):
|
|||||||
|
|
||||||
# lock and make a copy of the frame
|
# lock and make a copy of the frame
|
||||||
with self.frame_lock:
|
with self.frame_lock:
|
||||||
frame = self.shared_frame.copy().astype('uint8')
|
frame = self.shared_frame.copy()
|
||||||
frame_time = self.frame_time.value
|
frame_time = self.frame_time.value
|
||||||
|
|
||||||
# add the frame to recent frames
|
# add the frame to recent frames
|
||||||
@ -90,6 +92,161 @@ class FrameTracker(threading.Thread):
|
|||||||
if (now - k) > 2:
|
if (now - k) > 2:
|
||||||
del self.recent_frames[k]
|
del self.recent_frames[k]
|
||||||
|
|
||||||
# wait for the global motion flag to change
|
def get_frame_shape(rtsp_url):
|
||||||
with self.motion_changed:
|
# capture a single frame and check the frame shape so the correct array
|
||||||
self.motion_changed.wait()
|
# size can be allocated in memory
|
||||||
|
video = cv2.VideoCapture(rtsp_url)
|
||||||
|
ret, frame = video.read()
|
||||||
|
frame_shape = frame.shape
|
||||||
|
video.release()
|
||||||
|
return frame_shape
|
||||||
|
|
||||||
|
def get_rtsp_url(rtsp_config):
|
||||||
|
if (rtsp_config['password'].startswith('$')):
|
||||||
|
rtsp_config['password'] = os.getenv(rtsp_config['password'][1:])
|
||||||
|
return 'rtsp://{}:{}@{}:{}{}'.format(rtsp_config['user'],
|
||||||
|
rtsp_config['password'], rtsp_config['host'], rtsp_config['port'],
|
||||||
|
rtsp_config['path'])
|
||||||
|
|
||||||
|
class Camera:
|
||||||
|
def __init__(self, name, config, prepped_frame_queue, mqtt_client, mqtt_prefix):
|
||||||
|
self.name = name
|
||||||
|
self.config = config
|
||||||
|
self.detected_objects = []
|
||||||
|
self.recent_frames = {}
|
||||||
|
self.rtsp_url = get_rtsp_url(self.config['rtsp'])
|
||||||
|
self.regions = self.config['regions']
|
||||||
|
self.frame_shape = get_frame_shape(self.rtsp_url)
|
||||||
|
self.mqtt_client = mqtt_client
|
||||||
|
self.mqtt_topic_prefix = '{}/{}'.format(mqtt_prefix, self.name)
|
||||||
|
|
||||||
|
# compute the flattened array length from the shape of the frame
|
||||||
|
flat_array_length = self.frame_shape[0] * self.frame_shape[1] * self.frame_shape[2]
|
||||||
|
# create shared array for storing the full frame image data
|
||||||
|
self.shared_frame_array = mp.Array(ctypes.c_uint8, flat_array_length)
|
||||||
|
# create shared value for storing the frame_time
|
||||||
|
self.shared_frame_time = mp.Value('d', 0.0)
|
||||||
|
# Lock to control access to the frame
|
||||||
|
self.frame_lock = mp.Lock()
|
||||||
|
# Condition for notifying that a new frame is ready
|
||||||
|
self.frame_ready = mp.Condition()
|
||||||
|
# Condition for notifying that objects were parsed
|
||||||
|
self.objects_parsed = mp.Condition()
|
||||||
|
|
||||||
|
# shape current frame so it can be treated as a numpy image
|
||||||
|
self.shared_frame_np = tonumpyarray(self.shared_frame_array).reshape(self.frame_shape)
|
||||||
|
|
||||||
|
# create the process to capture frames from the RTSP stream and store in a shared array
|
||||||
|
self.capture_process = mp.Process(target=fetch_frames, args=(self.shared_frame_array,
|
||||||
|
self.shared_frame_time, self.frame_lock, self.frame_ready, self.frame_shape, self.rtsp_url))
|
||||||
|
self.capture_process.daemon = True
|
||||||
|
|
||||||
|
# for each region, create a separate thread to resize the region and prep for detection
|
||||||
|
self.detection_prep_threads = []
|
||||||
|
for region in self.config['regions']:
|
||||||
|
self.detection_prep_threads.append(FramePrepper(
|
||||||
|
self.name,
|
||||||
|
self.shared_frame_np,
|
||||||
|
self.shared_frame_time,
|
||||||
|
self.frame_ready,
|
||||||
|
self.frame_lock,
|
||||||
|
region['size'], region['x_offset'], region['y_offset'],
|
||||||
|
prepped_frame_queue
|
||||||
|
))
|
||||||
|
|
||||||
|
# start a thread to store recent motion frames for processing
|
||||||
|
self.frame_tracker = FrameTracker(self.shared_frame_np, self.shared_frame_time,
|
||||||
|
self.frame_ready, self.frame_lock, self.recent_frames)
|
||||||
|
self.frame_tracker.start()
|
||||||
|
|
||||||
|
# start a thread to store the highest scoring recent person frame
|
||||||
|
self.best_person_frame = BestPersonFrame(self.objects_parsed, self.recent_frames, self.detected_objects)
|
||||||
|
self.best_person_frame.start()
|
||||||
|
|
||||||
|
# start a thread to expire objects from the detected objects list
|
||||||
|
self.object_cleaner = ObjectCleaner(self.objects_parsed, self.detected_objects)
|
||||||
|
self.object_cleaner.start()
|
||||||
|
|
||||||
|
# start a thread to publish object scores (currently only person)
|
||||||
|
mqtt_publisher = MqttObjectPublisher(self.mqtt_client, self.mqtt_topic_prefix, self.objects_parsed, self.detected_objects)
|
||||||
|
mqtt_publisher.start()
|
||||||
|
|
||||||
|
def start(self):
|
||||||
|
self.capture_process.start()
|
||||||
|
# start the object detection prep threads
|
||||||
|
for detection_prep_thread in self.detection_prep_threads:
|
||||||
|
detection_prep_thread.start()
|
||||||
|
|
||||||
|
def join(self):
|
||||||
|
self.capture_process.join()
|
||||||
|
|
||||||
|
def get_capture_pid(self):
|
||||||
|
return self.capture_process.pid
|
||||||
|
|
||||||
|
def add_objects(self, objects):
|
||||||
|
if len(objects) == 0:
|
||||||
|
return
|
||||||
|
|
||||||
|
for obj in objects:
|
||||||
|
if obj['name'] == 'person':
|
||||||
|
person_area = (obj['xmax']-obj['xmin'])*(obj['ymax']-obj['ymin'])
|
||||||
|
# find the matching region
|
||||||
|
region = None
|
||||||
|
for r in self.regions:
|
||||||
|
if (
|
||||||
|
obj['xmin'] >= r['x_offset'] and
|
||||||
|
obj['ymin'] >= r['y_offset'] and
|
||||||
|
obj['xmax'] <= r['x_offset']+r['size'] and
|
||||||
|
obj['ymax'] <= r['y_offset']+r['size']
|
||||||
|
):
|
||||||
|
region = r
|
||||||
|
break
|
||||||
|
|
||||||
|
# if the min person area is larger than the
|
||||||
|
# detected person, don't add it to detected objects
|
||||||
|
if region and region['min_person_area'] > person_area:
|
||||||
|
continue
|
||||||
|
|
||||||
|
self.detected_objects.append(obj)
|
||||||
|
|
||||||
|
with self.objects_parsed:
|
||||||
|
self.objects_parsed.notify_all()
|
||||||
|
|
||||||
|
def get_best_person(self):
|
||||||
|
return self.best_person_frame.best_frame
|
||||||
|
|
||||||
|
def get_current_frame_with_objects(self):
|
||||||
|
# make a copy of the current detected objects
|
||||||
|
detected_objects = self.detected_objects.copy()
|
||||||
|
# lock and make a copy of the current frame
|
||||||
|
with self.frame_lock:
|
||||||
|
frame = self.shared_frame_np.copy()
|
||||||
|
|
||||||
|
# convert to RGB for drawing
|
||||||
|
frame = cv2.cvtColor(frame, cv2.COLOR_BGR2RGB)
|
||||||
|
# draw the bounding boxes on the screen
|
||||||
|
for obj in detected_objects:
|
||||||
|
vis_util.draw_bounding_box_on_image_array(frame,
|
||||||
|
obj['ymin'],
|
||||||
|
obj['xmin'],
|
||||||
|
obj['ymax'],
|
||||||
|
obj['xmax'],
|
||||||
|
color='red',
|
||||||
|
thickness=2,
|
||||||
|
display_str_list=["{}: {}%".format(obj['name'],int(obj['score']*100))],
|
||||||
|
use_normalized_coordinates=False)
|
||||||
|
|
||||||
|
for region in self.regions:
|
||||||
|
color = (255,255,255)
|
||||||
|
cv2.rectangle(frame, (region['x_offset'], region['y_offset']),
|
||||||
|
(region['x_offset']+region['size'], region['y_offset']+region['size']),
|
||||||
|
color, 2)
|
||||||
|
|
||||||
|
# convert back to BGR
|
||||||
|
frame = cv2.cvtColor(frame, cv2.COLOR_RGB2BGR)
|
||||||
|
|
||||||
|
return frame
|
||||||
|
|
||||||
|
|
||||||
|
|
||||||
|
|
Loading…
Reference in New Issue
Block a user